Machine Learning in Translational Medicine
We investigate the potential of artificial intelligence in every aspect of our research and employ machine learning methods to improve clinical care protocols and enable novel treatment options.
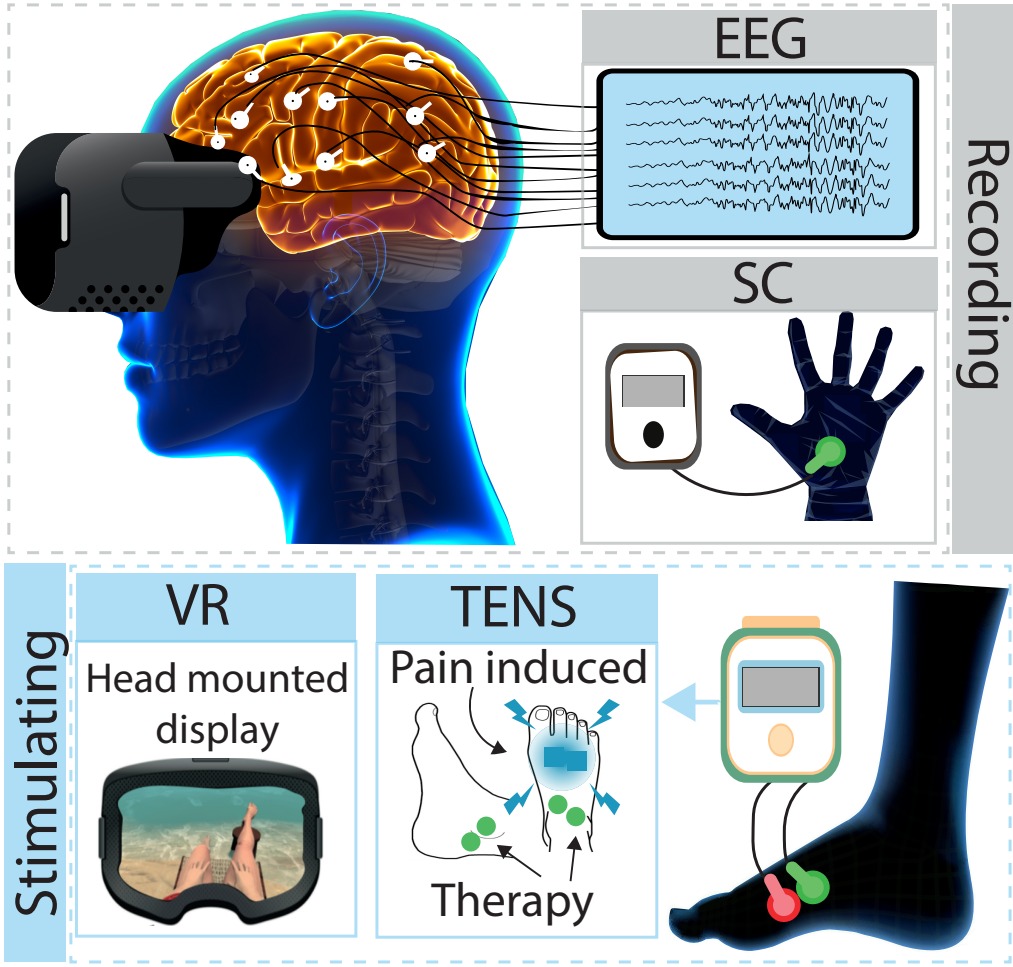
Reinforcement Learning for the calibration of neuroprosthetic legs
The identification of the electrical stimulation parameters for neuromodulation is a subject-specific and time-consuming procedure which presently mostly relies on the expertise of the user. Since the parameters of stimulation change over time (due to displacement of electrodes, skin status etc.), patients undergo recurrent, long calibration sessions, along with visits to the clinics, which are inefficient and expensive. To address this issue, we are developing automatized calibration systems for invasive and non-invasive sensory neuroprostheses. Our goal to provide a user-friendly, safe, efficient, and automatic calibration systems towards at-home use of personalized neuroprostheses. We are designing AI systems based on Bayesian Optimization and Reinforcement Learning, which are able to select neurostimulation parameters and electrodes to optimize the perceived sensation from the subject. See Neuroprosthetic Legs for additional information.
Unraveling the interplay between neurophysiological and psychological pain biomarkers
Chronic pain is an enormous economic and social burden, but its diagnosis relies solely on subjectively-reported pain scales. We leverage the use of advanced AI techniques such as multilevel, mixed-effect models on a highly-dimensional, hierarchical dataset collected from experimental pain procedures on more than 80 chronic pain patients to identify pain biomarkers and create an objective pain index that disentangles the physical versus emotional contributions in the reported pain level.
Closed-loop treatment of pain thanks to a Brain-Computer Interface and Transcutaneous Electrical Nerve Stimulation
The lack of reliable pain biomarkers and consequently non-personalized and ineffective pain treatments underlines the necessity for new therapies based on measurable and objective pain indicators. We developed a Brain Computer Interface (BCI) where advanced Machine Learning (ML) models are trained to recognize pain in real-time through Electroencephalography (EEG) and Skin Conductance (SC) recordings and accordingly deliver a multisensory therapy combining Transcutaneous Electrical Nerve Stimulation (TENS) and Virtual Reality (VR). Due to the intrinsic subjectivity and complexity of the pain experience, AI development is crucial for its detection. We aim to find the ML classifiers that maximize the detection performances of EEG and SC. This will help us take steps towards the use of AI in the development of real-time objective neurophysiological pain biomarkers to deliver individualized novel therapies.
Model-informed design of peripheral nerve electrodes and stimulation policies
Neurostimulation devices used in clinical care are poorly selective, meaning that the possibility to obtain desired physiological outcomes with tolerable side effects is very limited. Computational Models of the Peripheral Nervous System are invaluable tools to optimize electrode designs and stimulation policies. Through automatic optimization algorithms leveraging machine learning techniques, we develop novel electrode designs and stimulation policies. An important effort is being spent towards the development of closed-loop stimulation paradigms to account for the highly unsteady operation of implanted electrodes. Ultimately, we aim to fill the technological gap that inhibits the unbounded therapeutic potential of peripheral nerve stimulation with model-guided development of neuromodulation solutions.